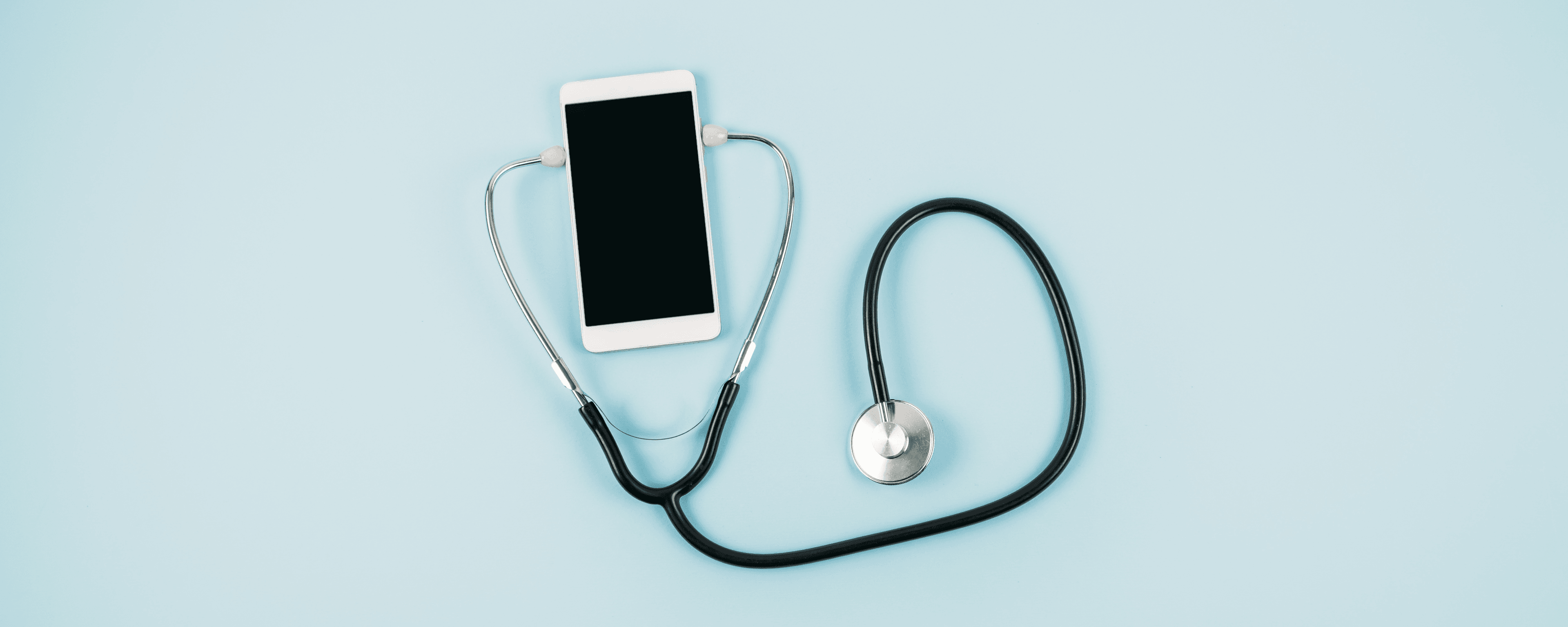
/articles/common-network-security-threats-and-vulnerabilities//articles/how-much-time-are-you-losing-to-technology-issues//articles/identity-and-access-management-vendors-how-to-choose-the-right-partner-for-your-organization//articles/mastering-incident-response-drills-best-practices-key-metrics//articles/prioritizing-patient-care-high-performance-for-telehealth-and-remote-patient-monitoring//articles/it-solutions-consulting-completes-acquisition-of-affinity-technology-partners-tennessee-based-it-service-provider//articles/green-it-strategies//articles/why-log-management-and-mdr-are-critical-for-small-to-midsize-businesses-smbs//articles/prepare-your-business-for-windows-10-is-nearing-end-of-life-step-by-step-guide//articles/2024-year-in-review//articles/it-solutions-celebrates-mission-2025-at-annual-sales-kick-off-in-las-vegas-nv//articles/7-major-cybersecurity-threats-businesses-face-today-and-how-to-mitigate-them//articles/benefits-and-challenges-of-cloud-adoption//articles/s-a-f-e-shopping-your-guide-to-smarter-social-media-shopping-this-holiday-season//articles/it-solutions-consulting-completes-acquisition-of-pace-technical-toronto-based-information-technology-service-provider//articles/haunted-by-cyber-risks-strengthen-business-cybersecurity//articles/understanding-cybersecurity-basics//articles/ensuring-data-security-in-healthcare-essential-cybersecurity-practices//articles/celebrating-30-years-of-excellence-a-qa-with-its-founder-and-ceo//articles/strong-authentication-methods-to-protect-your-business//articles/the-keys-to-proactive-cybersecurity//articles/five-reasons-to-implement-multi-factor-authentication-mfa//articles/siem-and-soc-the-cornerstones-of-modern-enterprise-security//articles/support-advisory-july-19-2024-microsoft-windows-outages//articles/bringing-microsoft-power-bi-and-dynamics-together-what-you-need-to-know//articles/unlocking-the-full-potential-of-microsoft-dataverse-for-teams-your-guide//articles/automation-enhancing-business-and-workflow-in-the-modern-era//articles/is-ai-getting-too-powerful-2024//articles/challenges-and-opportunities-in-data-mining-in-the-insurance-industry-2024//articles/navigating-itar-compliance-what-you-need-to-know-in-2024//articles/new-phishing-attacks-make-2fa-useless-updated//articles/how-to-make-microsoft-teams-your-default-for-meetings//articles/monitoring-employee-internet-usage-technology-ethics//articles/it-solutions-ranked-on-channel-futures-2024-msp-501-tech-industrys-most-prestigious-list-of-managed-service-providers-worldwide//articles/it-solutions-consulting-completes-acquisition-of-steady-networks-new-mexico-based-information-technology-service-provider//articles/why-is-my-phones-wi-fi-so-slow//articles/it-solutions-earns-spot-on-crns-2024-solution-provider-500-list//articles/6-digital-transformation-challenges-and-how-to-overcome-them//articles/it-solutions-announces-rebrand-experience-excellence//articles/3-updated-steps-to-secure-word-documents-with-a-password//articles/can-it-solutions-help-reduce-cyber-insurance-premiums//articles/how-to-blur-background-in-teams//articles/problems-with-two-factor-authentication-in-microsoft-365-2024//articles/get-to-know-our-client-success-team-nick-stebbins-veteran-and-client-success-trailblazer//articles/it-solutons-celebrates-success-innovation-sales-kickoff-2024//articles/it-solutions-consulting-recognized-on-crns-2024-msp-500-list//articles/what-are-the-best-ai-platforms-for-business//articles/2023-year-in-review//articles/it-solutions-consulting-completes-acquisition-of-the-network-pro-west-coast-based-it-and-security-service-provider//articles/cyber-security-awareness-training//articles/it-solutions-consulting-completes-acquisition-of-spade-technology-new-england-based-it-service-provider//articles/microsoft-word-2016-features-tips-and-tricks//articles/get-to-know-a-vcio-nathan-esposito-renaissance-man-client-champion//articles/it-solutions-recognized-most-promising-microsoft-solution-provider-2023-comprehensive-it-services//articles/digital-transformation-for-your-business//articles/telehealth-technology-it-strategies-patient-centered-care//articles/get-to-know-vcio-julissa-ortiz-client-advocate//articles/it-solutions-strengthens-healthcare-expertise-through-acquisition-of-umbrella-managed-systems-missouri-based-it-service-provider//articles/are-your-employees-your-biggest-security-threat//articles/acquisition-of-acropolis-technology-group//articles/how-to-ensure-your-business-is-compliant-with-cybersecurity-regulations//articles/article-when-to-update-your-software-and-why-it-matters//articles/get-to-know-a-vcio-paul-girgenti-music-aficionado-dedicated-it-leader//articles/article-vpns-protect-private-data-cybercriminals-third-parties//articles/pods-implementation//articles/2022-year-in-review//articles/sharepoint-extend-workforce-productivity-using-tools-you-already-have//articles/take-advantage-of-the-upgraded-it-solutions-client-portal-admin-edition//articles/debunking-common-cybersecurity-myths-in-the-workplace//articles/top-tips-to-identify-and-avoid-phishing-emails//articles/it-solutions-acquires-new-york-based-provider-of-it-support-cybersecurity-and-compliance-services-chips-technology-group//articles/take-advantage-of-the-upgraded-it-solutions-client-portal-user-edition//articles/article-it-solutions-digital-transformation-webinar-part-1//articles/it-solutions-recognized-among-top-msps-in-2022-rankings//articles/browsing-the-internet-tips-to-ensure-safety-when-clicking-a-link//articles/hr-compliance-webinar-the-info-you-need-to-keep-up-with-the-latest-laws//articles/article-data-loss-prevention-your-key-to-averting-a-data-disaster//articles/healthcare-compliance-in-2022-through-managed-technology-solutions//articles/a-best-place-to-work-2022//articles/dont-stress-over-it-hiring-our-services-and-support-cover-all-your-needs//articles/learning-management-system-training-em//articles/it-solutions-believes-in-giving-back-weve-given-nearly-9000-so-far//articles/anyone-without-strong-defenses-will-eventually-be-a-victim-dont-be-one-of-them//articles/article-no-better-time-to-review-tools-policies-and-confirm-your-level-of-protection//articles/cyber-insurance-webinar-top-cyber-insurance-gotchas-ensuring-the-lowest-premiums-2//articles/track-field-worker-time-manage-contacts-and-leverage-new-apps//articles/restore-a-previous-version-of-an-item-file-from-sharepoint-online-or-onedrive//articles/from-managing-contacts-in-the-field-to-online-reporting//articles/at-it-solutions-your-success-is-our-success-and-we-want-to-prove-it//articles/article-it-insights-two-factor-authentication//articles/shadow-it-the-unidentified-danger-lurking-inside-your-firm//articles/endpoint-security-and-much-more-comes-with-our-support-plans//articles/article-it-insights-siem-secure-incident-and-event-monitoring-system//articles/it-insights-security-posture//articles/it-insights-8-ways-to-protect-your-business-from-ransomware//articles/are-cybercriminals-stalking-your-firm-right-now-if-they-were-would-you-survive//articles/ransomware-is-exploding-are-you-prepared-to-protect-your-firm//articles/it-solutions-has-been-voted-a-top-philadelphia-workplace-for-the-fifth-time//articles/it-insights-staff-still-working-from-home-watch-this-to-assure-your-staffs-secure//articles/it-solutions-professional-services-moving-from-strategy-to-execution//articles/smiles-for-success-abington-jefferson-health//articles/top-tips-greater-productivity-with-microsoft-365-and-outlook-email//articles/the-its-fast-response-escalation-teams-on-the-spot-assistance-when-you-need-it-most//articles/tech-tip-working-with-files-sharepoint//articles/cross-functional-collaboration-microsoft-teams-great-product-keeps-getting-better//articles/combat-insider-threats-with-strong-defense-strategies//articles/where-digital-transformation-meets-business-transformation-cost-effective-real-world-examples//articles/are-data-breaches-your-top-business-concern-they-should-be//articles/cybercriminals-selectively-choosing-2021-attack-targets//articles/data-backup-and-recovery-why-your-business-cannot-survive-without-it//articles/kate-smith-director-of-client-strategy-strategy-game-is-1-for-this-eagles-fan//articles/tech-tip-install-microsoft-365-office-suite-multiple-devices-quickly-easily//articles/explore-how-cloud-technology-services-can-accelerate-your-business-advantage//articles/smiles-for-success-career-wardrobe//articles/sam-kramen-vcio-hound-lover-and-legal-industry-tech-extraordinaire//articles/still-running-microsoft-office-2010-its-time-to-upgrade-your-features-and-security-with-microsoft-365//articles/smiles-for-success-als//articles/it-solutions-ranked-among-worlds-most-elite-501-managed-service-providers//articles/microsoft-power-bi-the-business-intelligence-platform-driving-digital-transformation//articles/filemaker-19-overview-feature-summary//articles/tech-tip-adjusting-screen-display-resolution-or-magnification-for-ease-of-viewing//articles/it-solutions-to-host-launch-party-for-claris-filemaker-19//articles/security-as-a-service-webinar//articles/article-it-solutions-digital-transformation-webinar-part-2//articles/its-named-to-channele2e-top-100-vertical-market-msps-2020-edition//articles/tips-for-business-continuity-planning//articles/security-concerns-for-the-remote-workforce//articles/remote-team-collaboration//articles/smiles-for-success-home//articles/crn-2020-award-2//articles/crn-2020-award//articles/smiles-for-success-vmc//articles/25-year-anniversary//articles/its-acquires-securelement//articles/microsoft-365-admin-security-threat//articles/smiles-for-success-chop//articles/office-365-migration-mistakes-2//articles/office-365-migration-mistakes//articles/everything-you-need-to-know-about-microsoft-teams//articles/top-phishing-emails//articles/connected-to-community//articles/big-data-is-not-just-for-big-businesses//articles/sharepoint-and-custom-workflows//articles/sharepoint-benefits//articles/improve-productivity-with-microsoft-365-for-businesses//articles/top-msp-for-law-firms//articles/how-the-dark-web-impacts-business//articles/how-to-group-worksheets-in-microsoft-excel//articles/its-nearcloud-video//articles/take-your-network-security-to-the-next-level//articles/top-6-data-loss-prevention-tips-for-businesses-it-solutions//articles/signature-capture-feature-improvements-2//articles/seven-must-have-layers-of-protection-2//articles/seven-must-have-layers-of-protection//articles/10-telling-signs-its-time-for-a-new-website//articles/its-referral-spring-sprint//articles/material-design-angular-material-as-an-application-of-material-design-lite//articles/why-responsive-web-design-is-good-for-business//articles/entity-framework-database-first-versus-code-first//articles/8-productivity-tools-for-developing-custom-business-solutions//articles/five-windows-10-tricks-you-should-know//articles/5-steps-to-a-working-website-using-entity-framework-code-first//articles/microsoft-office-2016-quick-start-guide//articles/how-to-inspect-and-diagnose-web-errors-with-fiddler2//articles/transform-images-into-editable-documents-with-ms-office-lens-app//articles/windows-10-overview//articles/use-excels-paste-special-feature-for-advanced-functionality//articles/its-helps-rebuild-local-home-for-family-in-need//articles/it-solutions-launches-new-security-offering-for-comprehensive-data-protection//articles/understanding-usb-security-risks//articles/windows-server-2003-end-of-life//articles/eight-tips-for-secure-holiday-shopping//articles/forward-multiple-texts-at-once-on-your-iphone//articles/e-cycle-and-help-reduce-waste-and-hazardous-materials-in-your-community//articles/understanding-how-security-compliance-impacts-your-business//articles/how-to-create-a-poll-in-microsoft-outlook//articles/improve-internal-processes-with-custom-software-development//articles/3-multitasking-features-in-windows-to-maximize-your-screen-space//articles/career-wardrobe-selected-for-100000-technology-makeover//articles/update-cloud-giveaway-winner-announcement//articles/common-viruses-and-healthy-habits-to-avoid-them//articles/data-backup-and-disaster-recovery-plan//articles/10-telling-signs-youre-ready-for-the-cloud//articles/using-custom-animations-and-transitions-in-powerpoint//articles/it-solutions-lends-a-helping-hand-to-the-local-community-with-habitat-for-humanity//articles/quick-tip-how-to-use-field-codes-in-microsoft-word-2010-2//articles/quick-tip-how-to-use-field-codes-in-microsoft-word-2010//articles/it-solutions-volunteers-with-habitat-for-humanity-to-help-build-local-home//articles/nearcloud-is-here//articles/quick-tip-6-ways-to-ensure-your-email-gets-read//articles/5-keyboard-hacks-to-make-your-life-easier//articles/3-pc-ways-to-get-rid-of-an-old-pc//articles/how-to-use-bootstrap-framework-to-quickly-create-a-mobile-friendly-website//case-studies/new-england-construction-firm//case-studies/aalanco-service-corp-hvac-business//case-studies/control-systems-compliance-company//case-studies/saint-anthonys-health-center//case-studies/fort-washington-based-law-firm//case-studies/entertainment-construction-and-supply-company//case-studies/dann-dorfman-herrell-and-skillman//case-studies/medical-device-company//case-studies/hammond-shinners//case-studies/sher-shabsin-p-c//case-studies/byron-carlson-petri-kalb-llc//case-studies/beverly-farm-foundation//case-studies/plasma-services-group//case-studies/shannon-global-energy-solutions//case-studies/liberty-seafood//case-studies/clincierge//case-studies/microsoft-office-365-success-story//case-studies/strategic-banking-partners//case-studies/ddp-roofing//case-studies/lasalle//case-studies/assurancemd//case-studies/murray-devine-valuation-advisors//case-studies/rutgers-university-health-professions-office//case-studies/computer-measurement-group//case-studies/rainbow-sales//case-studies/the-ems-energy-institute-at-penn-state//case-studies/independence-cryogenic-engineering//case-studies/garfield-park-academy//case-studies/dws-printing//case-studies/cto-world-entertainment//case-studies/center-for-disabilities-studies-university-of-delaware//case-studies/cater-to-you//case-studies/sapling-inc//case-studies/pennsylvania-horticultural-society//case-studies/paramount-homes//case-studies/millennium-medical//case-studies/first-state-orthopaedics//case-studies/family-support-services//case-studies/limbaugh-construction-co-inc//case-studies/bradford-national-bank//case-studies/byerly-rv-center//case-studies/p-j-fitzpatrick//case-studies/glendale-chrysler-jeep-dodge-ram//case-studies/village-of-glen-carbon//case-studies/genovese-jewelers//case-studies/simmons-hanly-conroy//guides/dont-wait-migrate-with-its-your-guide-to-windows-10-eol//guides/the-growing-threat-cyberattacks-on-small-and-medium-sized-businesses//guides/empowering-businesses-with-integrated-cybersecurity//guides/penetration-testing-a-critical-safeguard-for-todays-smbs//guides/what-is-cmmc-compliance//guides/introduction-to-cloud-computing-for-businesses//guides/the-latest-chatgpt-features-a-comprehensive-training-by-it-solutions//guides/mastering-sharepoint-becoming-a-champion-of-your-organizations-digital-workspace//guides/mastering-microsoft-365-copilot-enhancing-productivity-with-ai//guides/where-are-my-files-navigating-onedrive-sharepoint-and-teams//guides/how-to-quickly-find-recover-word-documents//guides/what-is-aiaas-and-how-can-it-help-your-business//guides/telehealth-technology-what-do-you-need-to-get-started//guides/cyber-liability-insurance-what-should-you-expect-to-pay//guides/security-risk-assessments-why-your-business-needs-one//guides/cyber-liability-insurance-a-professional-guide-to-protect-your-business//guides/combatting-the-growing-frequency-of-ransomware-attacks-on-financial-services-organizations//guides/protecting-mobile-devices-and-application-data-with-microsoft-365//guides/how-to-create-fillable-forms-in-microsoft//mergers-acquisitions/top-3-msp-ma-insights-from-2025//mergers-acquisitions/to-integrate-or-not-to-integrate//mergers-acquisitions/effective-communication-strategy-drives-merger-acquisition-deal-success//mergers-acquisitions/four-key-metrics-msps-need-to-measure-acquisition-integration-success//mergers-acquisitions/why-most-ma-transactions-never-meet-synergy-expectations//mergers-acquisitions/drafting-letter-of-intent-loi-key-components-best-practices//mergers-acquisitions/strategies-for-msp-acquisition-finalize-buyer-seller-arrangements//mergers-acquisitions/business-acquisitions-deep-dive-into-due-diligence//mergers-acquisitions/what-to-expect-leading-up-to-loi-msp-merger-acquisition//mergers-acquisitions/bolt-on-blunder-msps-cautious-of-buying-small-competitors//mergers-acquisitions/seller-considerations-what-every-msp-owner-should-consider-when-exiting//mergers-acquisitions/how-to-increase-business-valuation-beyond-multiples//mergers-acquisitions/ma-deal-structures-is-an-earnout-the-best-solution-for-buyers-or-sellers//mergers-acquisitions/first-100-days-crafting-successful-merger-acquisition-integration-roadmap/
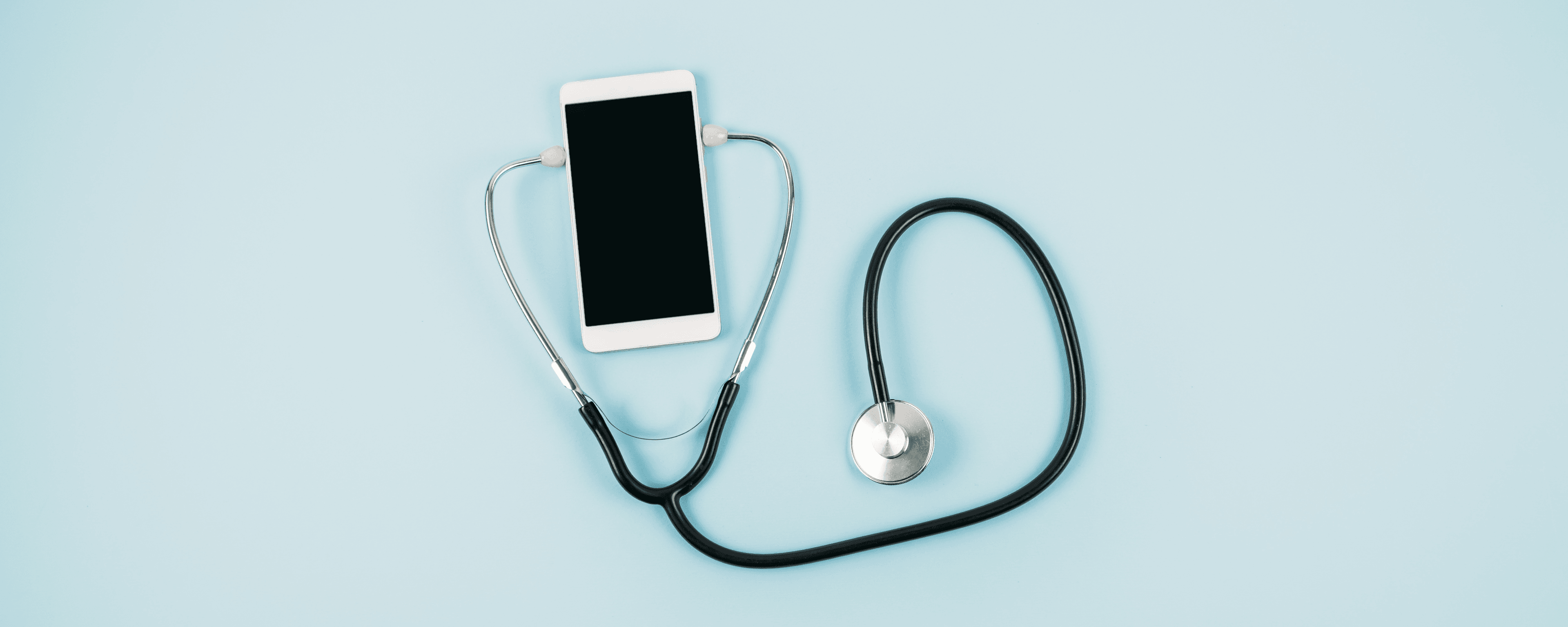